How scenario aggregation can improve risk management
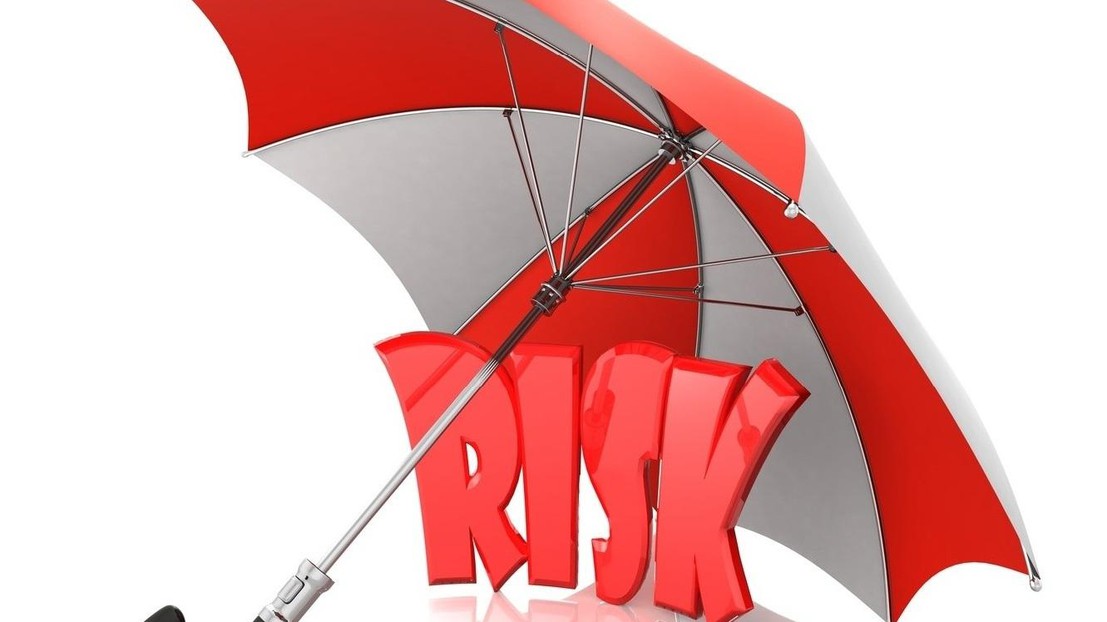
© 2015 Fotolia
Balancing conflicting interests can make risk management a challenge for organisations. Damir Filipovic and Mathieu Cambou show how external views on risk scenarios can be combined, and then used to fine tune internal risk models, providing a better perspective on risk.
When the global banking system almost unravelled in 2008, governments vowed to take new measures, and strengthen the existing regulatory framework, to help prevent a financial meltdown occurring in the future. Some of those measures involved better risk management. This included, for example, the use of risk modelling to gauge the ability of financial firms, such as banks and insurers, to withstand losses arising from specific events and allow them to put in place adequate capital buffers accordingly. Yet, despite the best intentions of governments and regulators, this kind of risk management is only as effective as the risk modelling methodology it is based on.
It is essential, therefore, that these models are tested and the methodology challenged. For example, the risk models used are often concerned with circumstances at the extremes (the tail) of both potential losses and probability. Analysing and understanding these extremes is difficult, any improvement in our ability to do this is welcome. This is an area that Damir Filipovic and Mathieu Cambou from the École Polytechnique Fédérale de Lausanne offer an innovative take on in their paper "Model Uncertainty and Scenario Aggregation".
Regulators demand a rigorous approach to risk management. Firms may be required to calculate capital solvency margins with 99.5 per cent confidence, in other words factoring in the worst possible situation likely to occur in a 200 year period. Yet the ability of financial firms to adequately factor in the complexity of potential risks that they face is limited. Looking back into the past to help anticipate the future is problematic, for example. Historical records are unlikely to cover such an extensive period. Plus, even if the data was available, the world is changing, risk profiles and probabilities alter over time.
One approach that can help fine tune risk models is scenario aggregation. Imagine a scenario where a market risk, such as a fall of 30 per cent or more in the S&P 500, is combined with another risk, such as a major earthquake in California, while at the same time interest rates go up by five per cent. Using the appropriate expertise, take a view on the probability of the event of this scenario occurring. Stack some scenarios up and compare the external view on these scenarios against a firm's internal risk model view on these scenarios. Then adjust the internal model accordingly.
While this may seem a sensible approach one obstacle is the difficulty in blending the more qualitative external aggregate scenario approach with the internal quantitative risk model. Filipovic and Cambou, however, have created a methodology that allows them to blend the two together and to satisfy a number of important criteria when doing so.
One criterion, for example, concerns the differing incentives of the regulator and the firm. While it is in the firm's interest to remain solvent and to maintain adequate financial buffers against potential losses, it also wants to create value for its shareholders and where possible maximise profits. The regulator, however, is concerned with both protecting a variety of stakeholders from potential losses, as well as managing systemic risk and preventing contagion. The regulator is more likely to take a conservative view.
Filipovic and Cambou ensure that their method does not penalise models with additional capital requirements if a scenario aggregation exercise reveals that the existing internal risk model is already sufficiently conservative. It also keeps any increases in the capital requirement to the minimum necessary to reflect any discrepancy between the external view and the results produced by the internal risk model.
For the authors' approach to be useful it must be relatively easy for firms to implement their method. Internal risk models are highly complex, it can take days to run an internal risk model and produce the appropriate capital requirement number, for example. The external scenario aggregation is a simpler exercise. Filipovic and Cambou's method ensures that any modification of the internal model to account for the views on the scenarios is kept to a minimum. It is also designed to allow firms to implement it relatively easily with minimum disruption.
Finally, the impact of different scenarios on firms will vary according to numerous factors. In the case of an insurance company, for example, (while Filipovic and Cambou's research focused on insurance it is equally applicable to banking, and other corporate risk management) it may depend on the type of insurance the firm underwrites, and where those risks are located. The approach devised by Filipovic and Cambou discriminates between companies depending on their particular vulnerability to the specific external risks, rather than assuming the potential impact on each firm will be identical.
Taken together these qualities make Filipovic and Cambou's method a valuable addition to robust risk management in financial firms. When setting capital safeguards, it allows regulators and firms a more precise view on the optimal balance between the interests of shareholders and society. And, hopefully if used widely may well help avoid a future global financial crisis.
Model Uncertainty and Scenario Aggregation by Mathieu Cambou, EPFL, Institute of Mathematics, and Damir Filipovic, Swiss Finance Institute Professor, and Swissquote Chair in Quantitative Finance and Head of the SFI at EPFL, forthcoming in 'Mathematical Finance'.