WISE 2014 Best Paper Award for Alexandra Olteanu and team
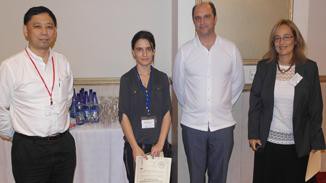
The program committee of WISE 2014 has awarded Alexandra Olteanu, Anne-Marie Kermarrec and Karl Aberer the Best Paper Award for their paper titled "Comparing the Predictive Capability of Social and Interest Affinity for Recommendations".
Alexandra Olteanu (EPFL), Anne-Marie Kermarrec (Inria) and Karl Aberer (Professor at the IC School) won the Best Paper at the 15th international Conference on Web Information System Engineering (WISE 2014). WISE is a peer-reviewed International conferences series which has so far established a solid community which aims at high quality research work and it offers the ground for advancing and progressing efforts in Web information systems addressed topics and audiences. The aim of this 15th edition of the conference series on Web Information Systems Engineering is to provide an international forum for researchers, professionals, and industrial practitioners to share their knowledge in the rapidly growing area of Web technologies, methodologies and applications.
The paper that won the award is entitled "Comparing the Predictive Capability of Social and Interest Affinity for Recommendations".
This study tries to shed light on when and why social affinity (e.g., how well connected users are in a social graph), is a better predictor of users' taste than interest affinity (e.g., how similarly users rate or items are rated). In addition to debunking popular believes about collaborative filtering and social recommendation systems, we also show that when the basis of formulating connections among users stems from plain friendship, rather than from sharing interests, relying on social affinity leads to less precise recommendations. Further, items likeability and user selectiveness (e.g., how items are rated or users rate on average) are good predictors of the recommendation approaches performance.
In the process, we underline the importance of aggregating and reporting performance metrics according to each recommendation application specificities. We show that one cannot rely on global metrics to assess a given recommender performance not only across various datasets, but also within each dataset across users or items demographics. Even a slight change in the global average might hide important changes in the performance distribution across dataset demographics. One may thus need to understand and optimize the performance on a specific demographic subset depending on the application specifics (e.g., for a beer recommendation service, it might be more important to be accurate in the recommendations to experienced and, thus, harder to please users).
We make a case for hybrid approaches, that dynamically adapt as the system evolves and the properties of user and item change over time."
Further information on http://delab.csd.auth.gr/wise2014/news.php?item=19.