When robots learn from our mistakes
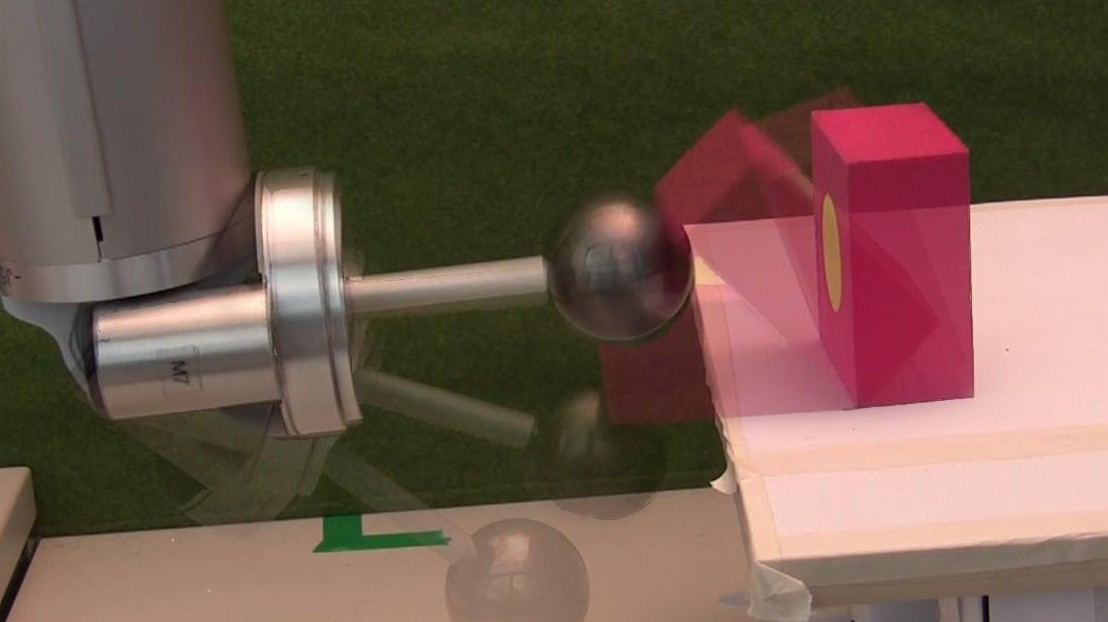
© 2011 EPFL
Robots typically acquire new capacities by imitation. Now, EPFL scientists are doing the inverse -- developing machines that can learn more rapidly and outperform humans by starting from failed or inaccurate demonstrations.
A robot, unblinking, impassive, observes. Its instructor wants it to learn how to put a balloon in a basket 20 meters away. As the researcher demonstrates this task, which is difficult for a human to accomplish, she systematically misses the basket. Isn’t the scientist just wasting her time?
Typically looked at simply as useless mistakes, failed demonstrations can, on the contrary, be opportunities to learn better, claim scientists from EPFL’s Learning Algorithms and Systems Laboratory (LASA). Their unusual point of view has led to the development of novel algorithms.
“We inversed the principle, generally accepted in robotics, of acquisition by imitation, and considered cases in which humans are inaccurate in certain tasks,” explains professor Aude Billard, head of LASA. “This approach allows the robot to go further, to learn more quickly and above all, outperform the human,” notes postdoctoral researcher Dan Grollman, who was recently awarded a “Best Paper Award” for an article on the subject presented at the International Conference on Robotics and Automation (ICRA), in Shanghai.
Grollman based his work on what he calls the “Donut as I do” theory. He developed an algorithm that tells the robot not to reproduce a demonstrator’s inaccurate gesture. The machine will use this input to avoid repeating the mistake and to search for alternative solutions. Thus the choice of the term “donut” – a play on the words “do not” and “donut.” The hole in the middle is the incorrect gesture, which must be excluded, and the surrounding dough represents the field of potential solutions to explore.
“We were inspired by the way in which humans learn,” explains Billard. “Children often progress by making mistakes or by observing others’ mistakes and assimilating the fact that they must not reproduce them.”
This way of learning things is a “real step forward,” says Grollman, who adds that, after all, “isn’t the real goal to make robots that can do things we can’t?”
Article: Daniel Grollman, Aude Billard, "Donut As I Do: Learning From Failed Demonstrations".